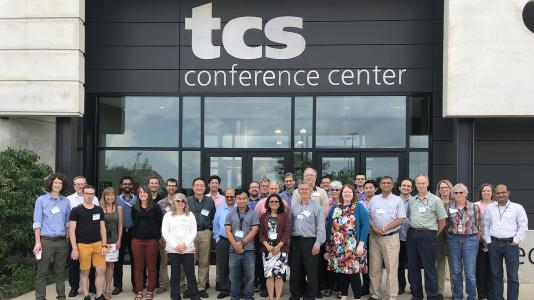
What can the latest trends in computer science reveal about the Earth’s physical, chemical and biological processes?
Scientists recently met at the U.S. Department of Energy’s (DOE) Argonne National Laboratory to answer that question. At the workshop, computational and natural scientists discussed opportunities for applying machine learning and geospatial statistics to challenging problems in environmental research.
Attendees spanned Argonne divisions, universities and governmental organizations such as the U.S. Forest Service and the U.S. Geological Survey. In a packed two-day program, attendees discussed recent developments in scientific machine learning and geospatial statistics and brainstormed how these rapidly developing technologies might enable new approaches to natural science research.
Participants found especially valuable the conversations between computational and natural science researchers, discussions that led to exciting ideas for collaborative research projects around the Earth’s system of physical, chemical and biological processes.
Machine learning is a form of artificial intelligence that builds on computer science, data science and statistics to give computers the ability to “learn.” Instead of adding data and programs into a computer to produce a designated output, machine learning allows computers to recognize patterns, both expected and unexpected, within data. These patterns can highlight environmental relationships in space and time that may unveil critical details about Earth’s condition.
“We humans are very good at detecting subtle patterns in data,” said Ian Foster, director of Argonne’s Data Science and Learning division. “But we’re limited in how much data we can consider. Machine learning can operate at speeds and scales that far exceed human capabilities, detecting patterns that humans would be unlikely to notice.”
Patterns that reveal spatio-temporal relationships are especially meaningful in the natural sciences. For example, important ecological measures such as contamination run-off levels and fertilizer usage can vary by location and time. The nexus of geostatistics and machine learning promises a better way to identify statistical relationships in such data that can reveal previously unsuspected relationships, while also mitigating individualized uncertainty.
The workshop’s presentations on hydrology, soil sciences, ecology and agriculture introduced attendees to topics across the natural sciences. Participants then discussed ways in which scientists can apply machine learning and statistics within various disciplines. Agriculturalists, for example, can analyze land use via remote sensing and satellite imagery. Ecologists can apply machine learning to classify and conclude how new species will respond to novel environments. Soil science specialists see machine learning as a way to determine soil functional type and improve land surface models, and hydrologists believe it will help them more efficiently clean up groundwater through a process called bioremediation.
“We sought to foster communication between two different groups — natural science researchers and computational and data scientists,” said Robin Graham, deputy associate laboratory director at Argonne.
Argonne scientists hope this communication will lead to new project proposals. Argonne’s immensely powerful supercomputers, including systems specialized for machine learning, provide a strong foundation for this type of work.
“National laboratories were originally formed to solve problems that require multiple disciplines to solve grand-challenge problems,” said Stefan Wild, deputy director of Argonne’s Mathematics and Computer Science division. “The candidate projects identified during this workshop harken back to those pioneering days in terms of the scale of the challenges and need for a confluence of broad expertise.”
This workshop was the first step toward a larger mission — moving toward a next generation of environmental science that applies the recent explosive developments in data science to large and diverse volumes of data to open up new vistas for research.
“We want to encourage domain scientists to look at machine learning as a new tool and really forge partnerships and collaborations to merge the two together,” said Cristina Negri, Argonne’s Environmental Science division director.
“Our knowledge and the science of machine learning and geostatistics is growing in leaps and bounds. But success depends on what resources, time, and effort we apply to make it work.”
Argonne National Laboratory seeks solutions to pressing national problems in science and technology by conducting leading-edge basic and applied research in virtually every scientific discipline. Argonne is managed by UChicago Argonne, LLC for the U.S. Department of Energy’s Office of Science.
The U.S. Department of Energy’s Office of Science is the single largest supporter of basic research in the physical sciences in the United States and is working to address some of the most pressing challenges of our time. For more information, visit https://energy.gov/science.