Leveraging computational chemistry to model the structural stability of polymers
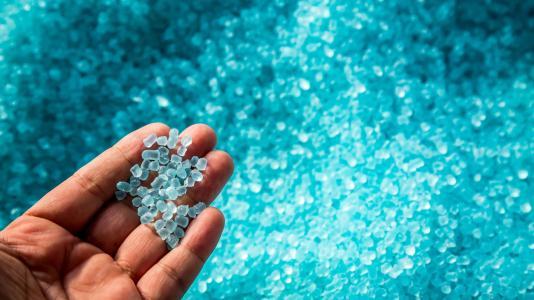
The Science
Knowing about a property called glass transition temperature (Tg) helps scientists understand how polymers and plastics work. Tg relates to the thermal energy required to transition between a hard like glass state to soft like rubber. It is a good approximation for stability. Scientists used two methods, machine learning (ML) and quantum chemistry (QC) based methods to predict Tg just by looking at the material’s structure. Investigating both ML and QC techniques provides insights into Tg model performance and applicability. The study showed both methods provided comparably accurate predictions of Tg. However, The QC-based method was more interpretable with fewer parameters.
The Impact
Scientists are working on creating new kinds of plastics and polymers that are less harmful and better for the environment. However, there are challenges. Developing these new materials requires the ability to predict what happens to them when they are thrown away and how toxic they are early in the design phase. Knowing information about their structure can help scientists estimate how fast the material will break down in the environment or degrade. Tg has been shown to greatly influence environmental degradation rates. It is a significant first step toward predicting these critical characteristics and developing screening tools to help design new materials.
Summary
Glass transition temperature (Tg) is important for understanding the physical and mechanical properties of a polymer material. It relates to the thermal energy required to transition between a hard glassy state and a soft rubbery one. Over the years, various models have been developed for predicting this thermal property at the molecular level to aid in designing new polymers.
This work builds on those efforts by utilizing both machine learning (ML) and quantum chemistry (QC) techniques to develop models that can predict Tg values from the molecular structure under different data availability scenarios and for a wide variety of polymer types. For the ML model, a graph convolutional network was used to map topological polymer features. This model was trained against a dataset of more than 7,500 Tg values and resulted in a root mean square error (RMSE) of 38.1 ℃, which is comparable to existing models on much narrower selections of polymer type. The QC-based regression model was trained on 83 Tg values and produced an RMSE of 34.5 ℃. This work demonstrated that while both model techniques produce accurate predictions and are suitable for different data availability scenarios, the QC-based regression model offered a more interpretable model framework with significantly less training data.